AI, Data Quality, and Hybrid/Decentralized Trials: Top Topics from Clinical Data Innovation Forum
What is the best way to achieve data quality? How are companies building a hybrid/centralized data strategy while decentralizing trials? How will artificial intelligence and machine learning shape the approach to clinical data science? The topics were key discussions among industry leaders and experts at the inaugural Veeva Clinical Data Innovation Forum in San Francisco. These are key themes and insights that highlight the opportunity for clinical data management.
Data quality remains a top challenge
Despite remarkable technological advancements in data collection, data quality continues to be a pressing concern, especially considering the increasing volume and variety of data sources in clinical research. The role of clinical data management is evolving to clinical data science, which encompasses aligning people, processes, and technology to to efficiently focus on meaningful, high-quality data. As one speaker noted, “The FDA doesn’t give you a ribbon for the cleanest data. They care that the data provided is meaningful, supports the study endpoints, and benefits the patient.”
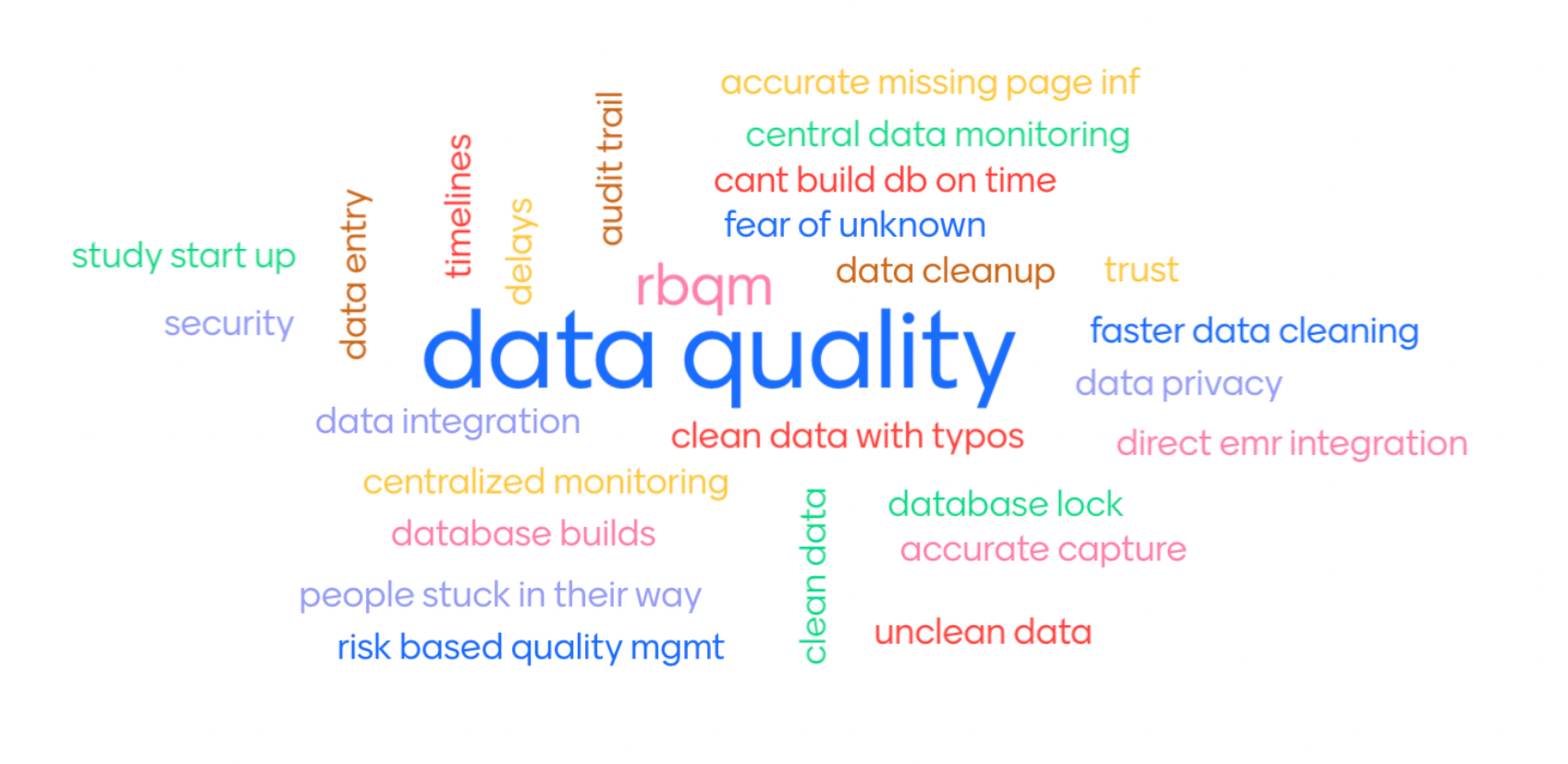
Responses to “What clinical data management challenges keep you up at night?”
Participants emphasized the need for data checks and validations, highlighting the importance of maintaining upstream data quality and applying aggregate quality checks downstream. They also underscored the significance of better protocol design and optimized technology utilization to minimize data quality risks during protocol amendments.
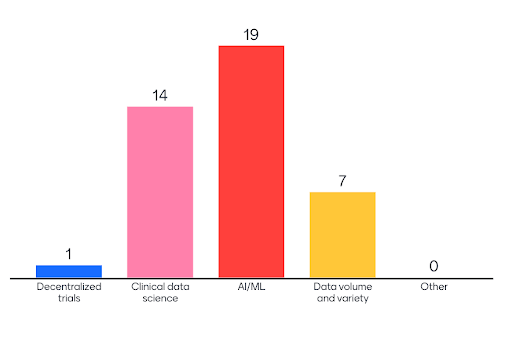
Responses to “Where do you see the biggest opportunities for innovation in clinical data management?”
The need for clinical data science
Organizations are transitioning from clinical data management to clinical data science, but the approaches may be different.There is an opportunity to collaborate across the industry to make this transition more effective. Data science has myriad roles to play throughout the organization, for example, helping to identify better sites or aiding in exploratory analysis. But as it pertains to data management, clinical data science should focus on the job to be done, accomplishing better quality data faster. To achieve this outcome, organizations need to ensure they have the right tools and processes to effectively aggregate data quickly and efficiently highlight potential quality issues on critical data. While organizations may need to upskill staff to maximize the value of their solutions, empowering their teams to focus on high-value initiatives should have a positive impact on retention.
The high potential of AI and ML
The potential of artificial intelligence (AI) and machine learning (ML) has the most potential to revolutionize clinical data management in the coming years. The consensus was that while AI and ML will likely automate repetitive tasks, they should be seen as tools that complement human expertise, enabling researchers to work smarter and faster.
Some of the use cases identified as being a good fit for AI/ML were:
- Generating plans/documentation, e.g., DMPs
- Medical writing/documentation
- Data quality checks against schema
- Instantiating study design
Embracing data centralization strategies
The importance of centralizing data as studies become more hybrid/decentralized was also a central theme. In the realm of clinical research, we encounter a variety of data sources that continue to grow. As studies become more hybrid and decentralized, the need to centralize data becomes even more critical.
Conference participants emphasized the need for robust data aggregation workflows that can bring together various data sources in a scalable manner and be able to efficiently distribute subsets to cross-functional stakeholders in desired formats. By establishing a strong data foundation, teams can leverage innovations such AI and ML to drive additional value.
Change management and adoption to maximize value
As new technologies and approaches are introduced in clinical data management, change management and collaboration emerged as crucial factors for successful adoption. Participants emphasized the importance of understanding the business requirements of different stakeholders and incorporating process improvements that maximize the value of change. “Don’t jump to solve. Focus on the why,” advised one of the speakers.
Sharing success stories and fostering collaboration within the industry were highlighted as essential strategies to drive the adoption of scalable solutions and facilitate the transition from clinical data management to clinical data science.
Join us at Veeva Summit R&D and Quality in Boston from September 13-14, 2023 to connect and ideate with over 1,500 of your life sciences peers.
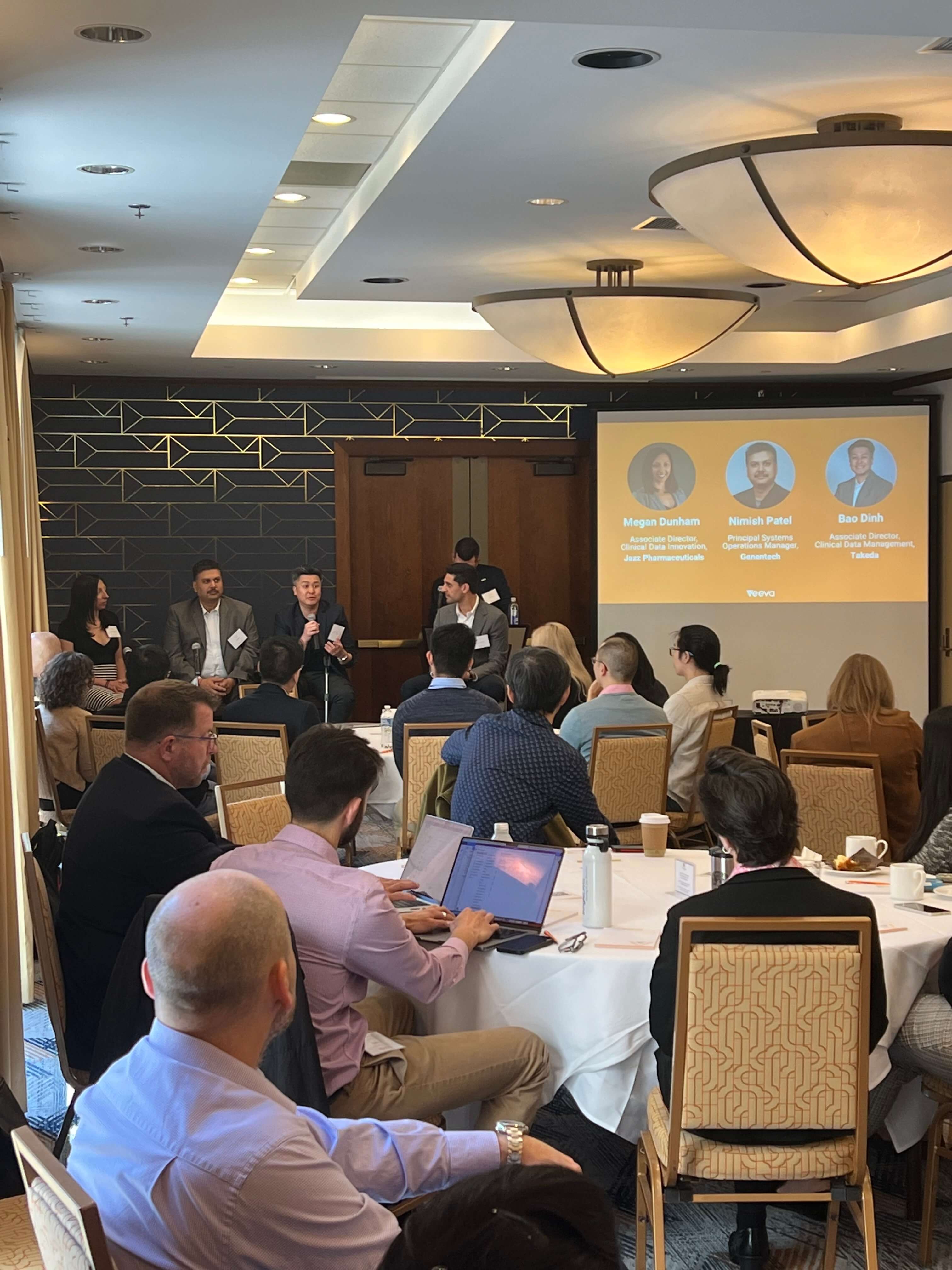