Is AI Hype Diluting Smart Automation’s Impact on CDM?
Sponsors and contract research organizations (CROs) are always on the lookout for methods to improve the speed, quality, cost, and safety of clinical trials processes. Recently, huge weight is being placed on artificial intelligence (AI) and machine learning (ML) as silver bullets to achieve these goals.
There are many use cases for AI/ML across the clinical trial lifecycle, from early drug development to patient recruitment, trial set-up, and data processing.1 But when asked about their clinical data workflows in a survey, only seven percent of industry leaders reported having integrated AI/ML across one or multiple applications.2 In addition, clinical data leaders ranked AI as an initiative with relatively low value and probability of success over the next two years [Figure 1]. Risk-based quality management (RQBM) and data science emerged as priorities for clinical data leaders who considered these areas most valuable and likely to succeed.
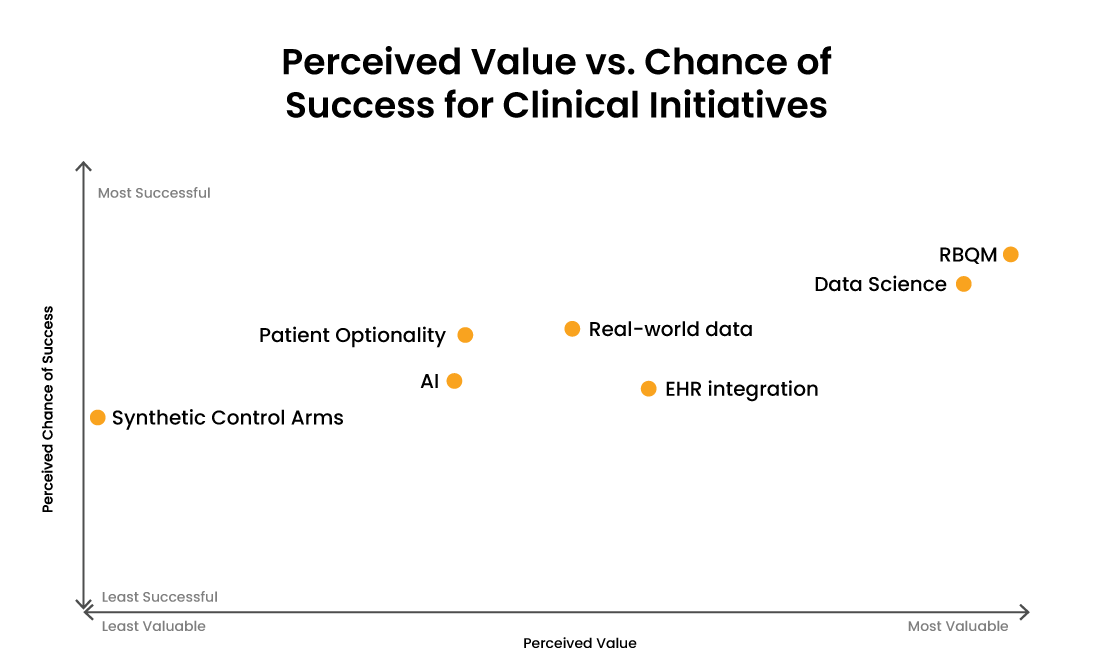
Figure 1: Combined responses to the survey question at three Clinical Data Innovation Forum sessions (New York, London, and Basel): “Which initiatives have the highest probability of success and the highest value in the next two years on a scale of 1-10?” RWD = Real-World Data.
Those responsible for clinical data management (CDM) face increasing pressure to carry out their tasks as quickly as possible. If we think about the famous technology adoption curve [Figure 2], AI/ML applications in the CDM space still fall into the ‘innovator’ or possibly ‘early adopter’ stages, which are characterized by relatively high costs coupled with lower value. So, although there’s significant potential for AI/ML to improve clinical trial efficiency, to date, the results have yet to match the hype.
Figure 2: The technology adoption curve: Defines five stages or markets that new high-tech products must move through to succeed.
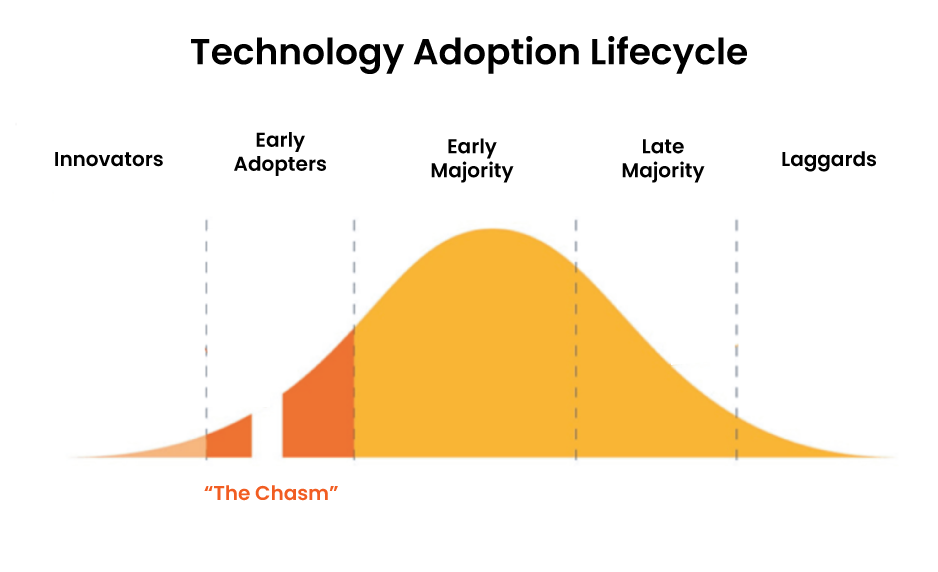
By contrast, algorithmic (non-AI) automation, which sits on the far right of the adoption curve, is adding significant value to CDM today. Algorithmic automation uses predefined rules, ranging from simple to complex, and executes those processes with great reliability and speed.
Using the right tools at the right time
Smart automation is an approach that emphasizes selecting the best automation technology to optimize efficiency while managing risk, which may be AI-based or algorithmic depending on the use case. There are several use cases where AI/ML tools could play a key role in the future by quickly detecting patterns, inconsistencies, and missing data. For example, the predictive power of ML could help forecast trial enrollment rates, identify risk factors that drive adverse events, and predict patient responses to treatments.3
While there are some early adopters of these technologies, we are yet to see their widespread implementation across clinical trials. A key reason for this is the inherent risk associated with AI’s behavior and its trustworthiness as a decision maker.
The effectiveness of an AI-based solution is all about context; low-context requests should be expected to produce low-context responses. Business problems such as identifying subjects that have a worsening condition, but have no associated adverse events (AEs) is a high-context challenge. It can most accurately be described using code or pseudocode as opposed to descriptive text. For AI to answer this question with the same degree of accuracy as rule-based automation, a human must review the output and provide the AI model with additional context in the form of feedback — a thumbs up or a thumbs down, for example. Because AI is a black-box solution, we can’t be sure how this training will impact its future output, and significant ongoing feedback is likely required to fine-tune its behavior. Ultimately, we can only be certain that AI is providing accurate responses by monitoring these responses.
For algorithmic automation, this unpredictability does not exist because an expert human has specified the expected behavior via code or pseudocode (high-context). Thereafter, no ongoing training is required and the tool can be trusted as a decision-maker.
Other risks of over-using AI/ML for clinical data management include a lack of:
- Consistency
Because AI’s logic is contained within the black box, we cannot be 100% sure what response an AI solution will provide to a given question or scenario. This potential lack of consistency requires ongoing human oversight and is a cause for concern for both regulators and the industry. - Transparency
We don’t always know why an AI model reaches a prediction or suggestion. But researchers rely on clear justifications of decisions, and patients must be able to understand results to trust these decisions. - Adaptability
Because AI models are trained using existing datasets, they might not be able to adapt to new data or populations, minimizing their transferability and scalability.4 - Regulatory guidance
The US Food and Drug Administration (FDA) is yet to issue regulatory guidelines for AI use in clinical research. The Artificial Intelligence Act (AIA), established on 1st August 2024, provides a common regulatory and legal framework for AI within the EU but does not specify its application to clinical research.
So, how can we continue to advance CDM without waiting for the industry to overcome these hurdles?
Utilizing a strong data foundation
Accelerating database lock in clinical research is a critical aspect of expediting study conclusions and decision-making. As a result, researchers and companies can develop therapeutic innovations and interventions faster.
A solution that is producing efficiency gains today involves pairing clinical data workbenches with algorithmic automation tools. Clinical data workbenches aggregate study data across all sources, including EDC, labs, and eCOA, and harmonize it in a central location. Algorithmic (or rule-driven) automation is a proven approach that provides up to 100% accuracy while minimizing human oversight.
Deploying algorithmic automation within a centralized data workbench enables low-risk, highly scalable automation use cases that aren’t possible with siloed data systems. This strategy has been shown to significantly reduce manual effort and redundant work in the areas of data review and cleaning.
An example of this automated workflow was presented by Graham Craig, data management director, GSK, at the 2024 EU R&D and Quality Veeva Summit. Craig’s team historically used more than 40 systems across data management. Their new end-to-end model, built on Veeva CDB and Vault EDC, automates clinical data management, from aggregation to reporting:
- Aggregation
A single clinical data workbench enables aggregation of all data sources through automated data ingestion, rather than working with many manual listings. - Cleaning
The clinical data workbench enables a current, holistic view and efficient oversight of the data cleaning process. This gives stakeholders an up-to-date view of the status of data cleaning. - Freezing and locking
Automated patient data freezing and locking within the EDC system allows data to be subset in unique ways based on the criteria, reduces the need for study data tabulation model (SDTM) review, and enables clinical study reports to be started quicker. - Data change extract reports
Identifying changes to data was a huge task before implementing EDC. Now the team can run a report and identify any changes at the subject, site, country, or study level. The extract functionality also enables a risk-based approach to audit trail review.
Read more about GSK’s workflow and results in this summary.
Maximizing the value of today’s technology
Technology that reduces the manual effort of managing clinical data is undoubtedly transforming trial efficiency. GSK provides an example of how clinical data workbenches like Veeva CDB can provide a centralized repository for trial data and are a key tool for supporting smart automation.
The innovators already implementing AI in the CDM space are crucial; our industry needs those with the resources and appetite for risk to help move our collective capabilities forward. But by deploying algorithmic automation through a clinical data workbench, we maximize efficiency gains today while gathering aggregated, cleaned clinical data to fuel future AI experiments.
Learn more about the benefits of automating clinical data flows.
1 Beaney, A. ‘SCOPE: AI in clinical trials is here to stay. How should it be used?’, Clinical Trials Arena, 2024.
2 eClinical Solutions, 2024 Industry Outlook Report
3 Walters, L. ‘Artificial Intelligence and Machine Learning in Clinical Data Management: Opportunities and Ethical Considerations’, PharmiWeb, 2024.
4 Chopra, H. et al., ‘Revolutionizing clinical trials: the role of AI in accelerating medical breakthroughs’, International Journal of Surgery, 2023.